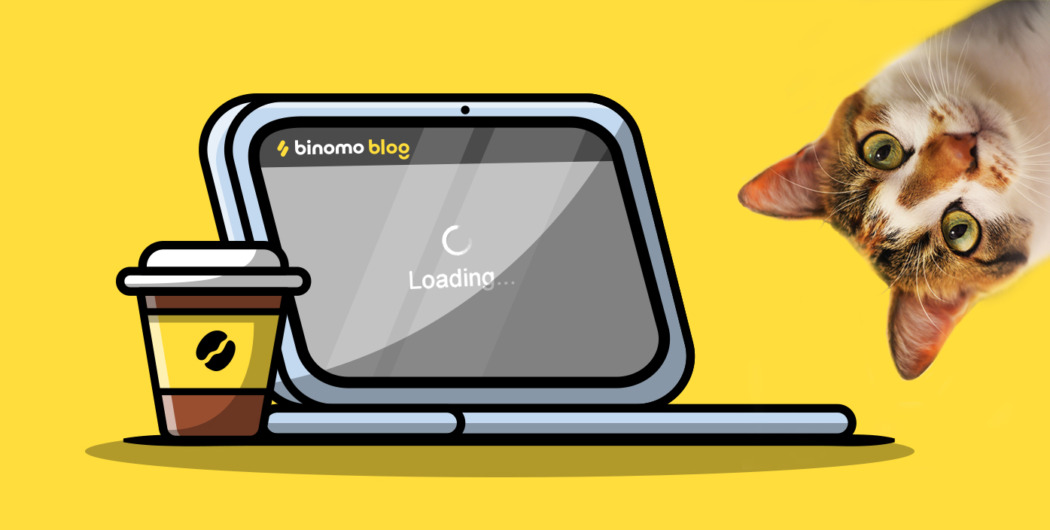
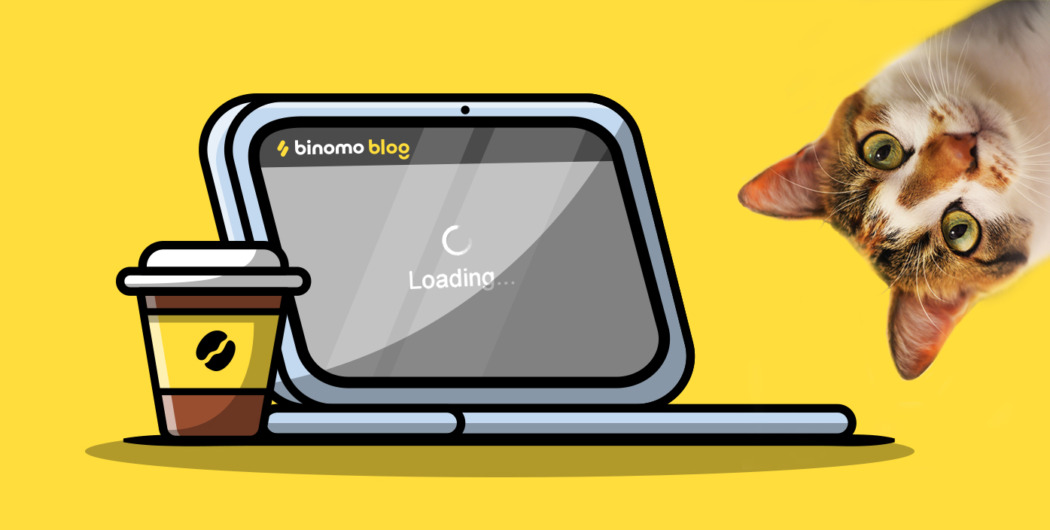
In 1905, Karl Pearson introduced kurtosis, a degree of curve. In this article, we discuss what kurtosis is, its types, and the calculation formula.
What is Kurtosis?
Kurtosis is a statistical measure. The use of kurtosis allows you to get a characteristic of a dataset.
The data plotted on a chart and furthest from the mean of the data forms tails on each side of the curve. Using kurtosis allows you to determine how much data is in the tails. A positive kurtosis indicates heavier tailedness and more peakedness. A negative kurtosis means a flat and lighter tail.
So, kurtosis provides information about the tail, helps to identify departure from normality, and helps compare. In other words, kurtosis is an indicator that quantifies the heaviness and thickness of the tail.
There are three types of kurtosis distribution: leptokurtic, mesokurtic, and platykurtic. They measure and describe the shape, tail, and peakness. In probability distribution, it is giving an opinion of a deviation of a distribution from a normal one, referred to as a bell shape. We will discuss them in more detail below.
Consider distributions with low and large kurtosis. In the first case, the distributions have less tail data than normally distributed data, pushing the bell curve’s tails away from the mean. In the second case, the distributions have more tail data, which brings the tails closer to the mean.
What information does kurtosis give investors? The high kurtosis of the return distribution shows that there used to be a lot of price fluctuations away from the average investment return. It turns out that, in this case, the investor may face sharp price fluctuations. This is called excess risk.
Understanding Kurtosis
Kurtosis is an indicator that shows the ratio of the distribution tails to the mean (the center of the distribution curve). For example, we have a histogram with some data that shows the peak of the bell. With normal kurtosis, most data is within three standard deviations of the mean. However, at high kurtosis, the tails extend further than three standard deviations of the normal bell distribution.
Kurtosis describes the shape of the tails of a distribution about its overall shape. Do not confuse it with a measure of the peakedness of a distribution; kurtosis measures “tail”, not “pointiness”. So, a distribution may have a low peak with a high kurtosis, and vice versa.
Formula and Calculation
Kurtosis characterizes a distribution’s relative flatness or peakedness compared with the normal one. A negative kurtosis indicates a relatively flat distribution; conversely, a positive kurtosis indicates a relatively peaked distribution.
There are various methods to calculate kurtosis, but the easiest way is to use the formula of Google Sheets or Excel. Let’s say you have the following data samples: 3, 4, 5, 2, 3, 4, 5, 6, 4, and 7 in cells A2 through A11 in your spreadsheet. In this case, use this formula to calculate kurtosis:
where:
n — the sample size;
xi — observations of the variable x;
x̄ — the mean of the variable x;
s — sample standard deviation.
Calculating kurtosis manually takes time, so we recommend using computer software. It’s even easier if you use the following Google Sheets or Excel kurtosis formula based on the data in cells A2 through A11:
=KURT(A2:A11)
Example
Below is the calculation of the kurtosis formula in the Excel worksheet.
Formula | Description | Result |
=KURT(A2:A11) | Kurtosis of the dataset above | -0.1517996372 |
The result is a kurtosis of -0.1518, indicating that the curve is platykurtic with lighter tails.
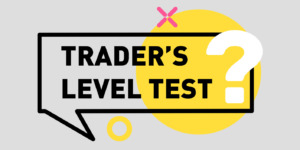
Types of Kurtosis
There are three categories of kurtosis that a dataset can display — mesokurtic, leptokurtic, and platykurtic. All measures of kurtosis are compared against a normal distribution curve.
What is a mesokurtic distribution?
In a mesokurtic distribution, outliers are neither highly rare nor highly frequent. It has a medium tail. For example, sometimes a female baby elephant is born weighing less than 180 or more than 240 pounds, with an average of 210 pounds.
It turns out that the characteristic of the extreme distribution value is similar to the characteristic of the normal distribution. In this case, kurtosis = 3 because normal distributions have kurtosis 3.
Investor Benefit — stocks with a mesokurtic distribution present a moderate level of risk.
Mesokurtic distribution example
Commonly, a female baby elephant weighs approximately 210 pounds at birth. In our example, the scientist is interested in the birth weight distribution of elephants. To do this, he requests data from reserves and zoos worldwide. As a result, he collects data on the birth weight of 400 female baby elephants.
These data are shown in the chart above. As you can see, the frequency distribution (gray bars) roughly corresponds to the normal distribution (green curve). Thus, the normal distribution is mesokurtic.
The scientist decides to calculate the kurtosis of the sample: it is 3.09, and the excess kurtosis is 0.09. Thus, he thinks that the distribution is mesokurtic.
What is a platykurtic distribution?
The term “platykurtic” is a Greek word, where “platús” means flat, and “kurtos” means bulging. It is a statistical term that is used to measure distribution.
With a platykurtic distribution, the kurtosis is less than with a normal distribution. In this case, kurtosis < 3 and excess kurtosis < 0.
It has a flatter and thinner tail, which indicates infrequent outliers. Sometimes, platykurtosis is referred to as negative kurtosis because excess kurtosis is negative.
Investor Benefit — stocks with a platykurtic distribution present a less-than-moderate level of risk.
Platykurtic distribution example
Here, we explain platykurtic with an example. A sociologist studies how many high school students aged 14 to 18 are fond of sports. The school has 400 students of the specified age.
This data is shown in the chart above. As you can see, the frequency distribution (gray bars) does not follow a normal distribution (green curve). However, they roughly correspond to a uniform distribution (purple curve). Thus, the uniform distribution is platykurtic.
The sociologist decides to calculate the kurtosis of the sample: it is 1.78, and the excess kurtosis is 1.22. Thus, he thinks that the distribution is platykurtic.
Note! On the graph, you can see in the extreme right and left parts of the distribution (tails) the space under the purple curve (uniform distribution) is thinner than under the green curve (normal distribution). These are the “thin tails”.
What is a leptokurtic distribution?
The term “leptokurtic” is a Greek word, where “leptós” means narrow, and “kurtos” means bulging. To remember the meaning of leptokurtic, imagine a jumping kangaroo with a fat tail.
The leptokurtic distribution means a lot of outliers, so it has a fat tail. It is more kurtotic than the normal distribution, so its kurtosis > 3 and excess kurtosis > 0. Sometimes, leptokurtosis is referred to as positive kurtosis because excess kurtosis is positive.
Leptokurtic distribution example
In our example, 4 astronomers are trying to measure the distance between Earth and Nu2 Draconis A, a blue star in the constellation Draco. Each measures the distance 100 times and sends the obtained information to a common dataset.
This data is shown in the chart above. As you can see, the frequency distribution (gray bars) does not follow a normal distribution (green curve). However, they roughly correspond to a Laplace distribution (blue curve). Thus, the Laplace distribution is leptokurtic.
The astronomers decide to calculate the kurtosis of the sample: it is 6.54, and the excess kurtosis is 3.54. Thus, they think that the distribution is leptokurtic.
Note! On the graph, you can see in the extreme right and left parts of the distribution (tails) the space under the blue curve (Laplace distribution) is slightly thicker than under the green curve (normal distribution). These are the “fat tails”.
Using Kurtosis
Investors use kurtosis to measure the investment risk of price volatility. It gauges the degree of volatility that the price of an investment has regularly experienced.
How can an investor use this data? A high kurtosis indicates two opposing factors since it can fluctuate in both ways: either a large positive or a large negative return. So, a high kurtosis of the distribution of returns may mean that investments will generate extreme returns from time to time.
Suppose the average price per share is $19.75. If this price fluctuated widely and often, the bell curve would have heavy tails (high kurtosis). Such tails show that the stock price fluctuates a lot, which should be considered when investing.
In contrast to a high kurtosis, a low kurtosis indicates a more predictable return profile, i.e., carries a lower risk of losing funds. To reduce risk and create a safer and less volatile portfolio, looking for investments with lower kurtosis is essential.
Kurtosis vs. Other Common Measurements
Kurtosis is a statistical measure that shows how much of the data in a probability distribution is centered around the mean versus the tails. Following are some commonly used kurtosis:
- Alpha gauges the excess returns relative to the benchmark index.
- Beta gauges the stock volatility relative to the broader market.
- The R-square gauges the percentage of portfolio movement the benchmark can explain.
- The Sharpe ratio compares return to risk.
Kurtosis measures the peak or flatness of the distribution, and Alpha and Beta measure the degree of asymmetry of the distribution.
The R-square is used to evaluate the accuracy of the regression model fit (in regression analysis) while kurtosis is used to describe the shape of the distribution (in descriptive statistics).
If we compare kurtosis and the Sharpe ratio, the first analyzes the distribution of the dataset, and the second evaluates the effectiveness of investments. Investors use the Sharpe ratio to see if the level of return they receive matches the level of risk incurred.
FAQs
Consider what questions investors are interested in about kurtosis.
What Is The Difference Between Skewness And Kurtosis?
Skewness measures the distribution’s relative symmetry about the mean. It is used to measure the data for symmetry. It may be positive, negative, and zero skewness. If the coefficient of skewness (CS) = 0, then the data is symmetric. In positive skewness, the tail is longer on the right-hand side. While in negative skewness, the tail is longer on the left-hand side.
On the other hand, kurtosis measures the tailedness or peakedness of a normal distribution. It shows that the dataset outliers the mean. It also has three types: leptokurtic (> 3), mesokurtic (= 3), and platykurtic (< 3). In skewness, we measure symmetry; in kurtosis, we measure lighter and heavier tails.
Why Is Kurtosis Important?
Kurtosis plays an important role in finance and investment. It is used to determine whether the dataset follows the normal distribution. The normal distribution is equal to 3. It helps to distinguish between different distributions. Like leptokurtic has a thin peak, mesokurtic is normal distribution, and platykurtic is probably flat.
What Is Excess Kurtosis?
Excess kurtosis compares the coefficients of a normal distribution and kurtosis. In a normal distribution, kurtosis is equal to three. Thus, the excess kurtosis will be greater than or less than three. In some models, a normal distribution equals zero, so kurtosis will be greater than or less than zero.
Excess kurtosis in investment is a tail risk or the probability of loss due to a rare event predicted by the probability distribution. If such events occur more often than the distribution indicates, the tails are called “fat”.
What Are The Uses Of Kurtosis In Data Analysis?
It describes the distribution of a data set. Kurtosis shows the extent to which the data set points of a particular distribution differs from those of a normal one. You can also use it to determine if a distribution contains extreme values.
The Bottom Line
Kurtosis is a statistical measure that describes the characteristics of a dataset. It shows how much probability distribution falls on the tails, not the center. It has various uses in fields like finance, economics, data analysis, and more. Investors can use it to get valuable data for future investment because it allows you to understand tail risk. However, always remember that it is essential to double-check the data received to avoid the risk of losing funds.